如何在焊接機器人進行設(shè)備預(yù)測性維護?
來源:http://niuniury.com/ 發(fā)布時間:2023-05-30 瀏覽次數(shù):0
工業(yè)焊接機器人機械,電氣系統(tǒng)復(fù)雜,工作區(qū)域大,運行速度快,因而無法準確預(yù)測在不同工況下有可能出現(xiàn)的所有危險,尤其在人工示教編程或者維護時,任何操作失誤和未知的系統(tǒng)缺陷都有可能造成設(shè)備損壞甚引發(fā)重大事故。那么如何在焊接機器人進行設(shè)備預(yù)測性維護?山東數(shù)控焊接設(shè)備廠家為您分析:
The mechanical and electrical systems of industrial welding robots are complex, with large working areas and fast operating speeds, making it difficult to accurately predict all the hazards that may occur under different working conditions. Especially during manual teaching programming or maintenance, any operational errors and unknown system defects may cause equipment damage or even major safety accidents. So how to perform predictive maintenance on welding robots? Shandong CNC welding equipment manufacturer analyzes for you:
預(yù)測性維護的分類
Classification of Predictive Maintenance
預(yù)測性維護可以分為基于設(shè)備機理和基于數(shù)據(jù)驅(qū)動預(yù)測兩種類型?;跈C理模型的預(yù)測是建立設(shè)備故障與機械動力學(xué)、熱力學(xué)和計量學(xué)等數(shù)學(xué)模型的關(guān)聯(lián)關(guān)系預(yù)測設(shè)備故障,而數(shù)據(jù)驅(qū)動模型則是通過大量數(shù)據(jù)的學(xué)習(xí)和訓(xùn)練,形成智能化的決策模型。
Predictive maintenance can be divided into two types: device mechanism based and data-driven prediction based. The prediction based on mechanism model is to establish the relationship between equipment failure and mathematical models such as mechanical dynamics, thermodynamics and metrology to predict equipment failure, while the data-driven model is to form an intelligent decision-making model through learning and training a large amount of data.
前者更適用于旋轉(zhuǎn)類設(shè)備,數(shù)據(jù)驅(qū)動模型更適用于復(fù)雜不確定系統(tǒng)和黑箱過程的預(yù)測和控制,數(shù)據(jù)驅(qū)動模型是基于經(jīng)驗數(shù)據(jù)統(tǒng)計關(guān)系或統(tǒng)計特征的預(yù)測和控制方法,其效果依賴于輸入數(shù)據(jù)的準確性和響應(yīng)頻率。
The former is more suitable for rotating equipment, while data-driven models are more suitable for prediction and control of complex uncertain systems and black box processes. Data-driven models are prediction and control methods based on empirical data statistical relationships or statistical features, and their effectiveness depends on the accuracy and response frequency of input data.
預(yù)測性維護的實施流程
Implementation process of predictive maintenance
01
01
數(shù)據(jù)獲取
Data acquisition
通過模擬仿真和傳感器測量獲得目標設(shè)備或系統(tǒng)的全壽命數(shù)據(jù)。
Obtain full life data of the target equipment or system through simulation and sensor measurement.
02
02
數(shù)據(jù)處理
data processing
包括數(shù)據(jù)預(yù)處理和特征提取,對數(shù)據(jù)進行過濾和整理,識別數(shù)據(jù)中工況信息,剔除非重要變量,通過特征提取的方法得到衰退特征,供模型訓(xùn)練使用。
This includes data preprocessing and feature extraction, filtering and organizing the data, identifying working condition information in the data, removing non important variables, and obtaining decay features through feature extraction methods for model training.
03
03
特征提取
feature extraction
刪除對任務(wù)無有用信息的屬性,對傳感器數(shù)據(jù)特征提取方法進行設(shè)計,建立基于傳感數(shù)據(jù)特征提取的計算機預(yù)測性維護模型,并進行對比實驗。
Delete attributes that have no useful information for the task, design feature extraction methods for sensor data, establish a computer predictive maintenance model based on sensor data feature extraction, and conduct comparative experiments.
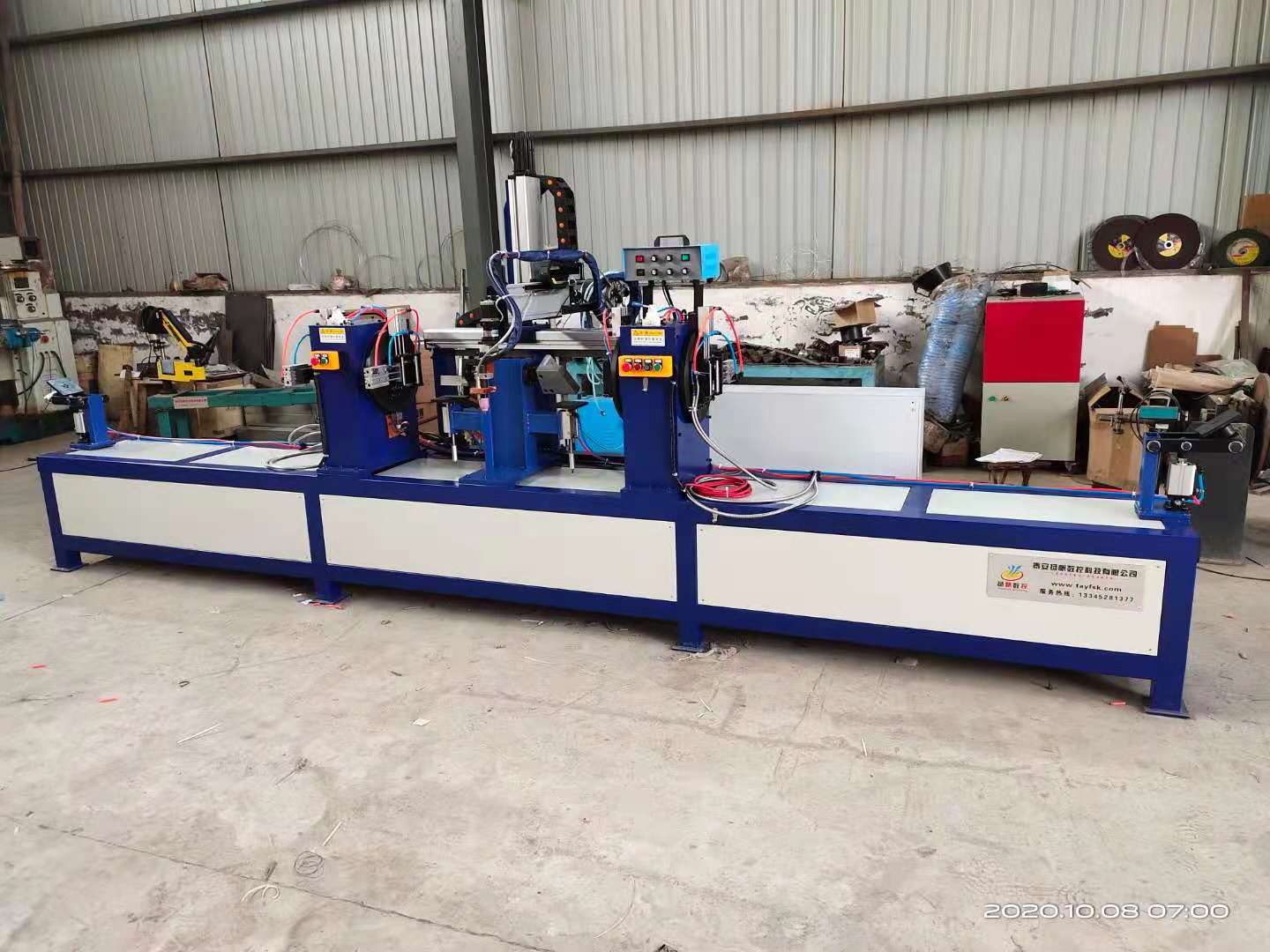
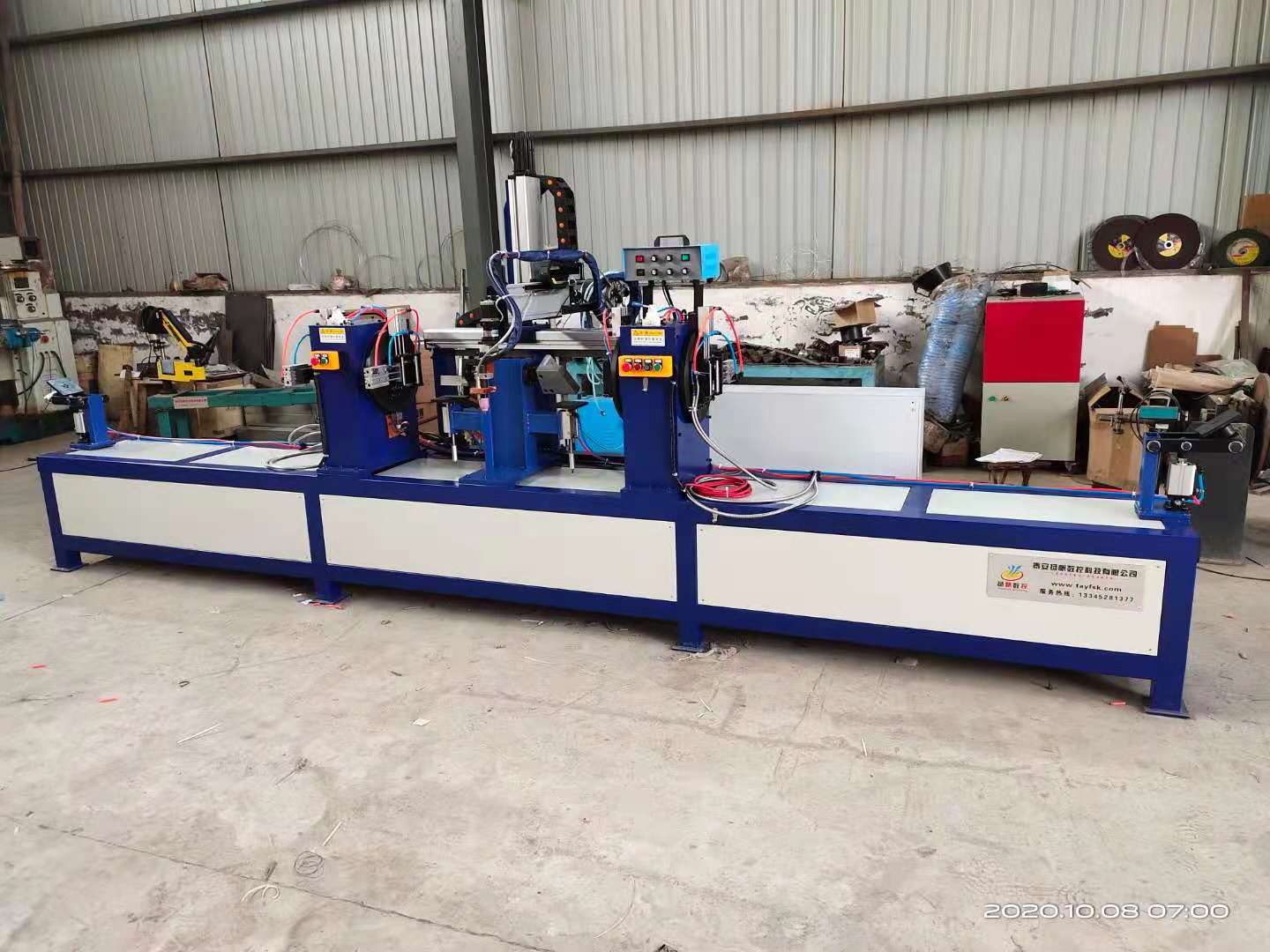
04
04
模型訓(xùn)練
model training
選擇適當(dāng)機器學(xué)習(xí)模型,利用經(jīng)處理后的全壽命數(shù)據(jù)進行訓(xùn)練,獲得在不同工況下可以對設(shè)備的故障進行準確預(yù)測或系統(tǒng)剩余壽命進行準確預(yù)測的模型。
Select appropriate machine learning models and train them using processed full life data to obtain models that can accurately predict equipment failures or system remaining life under different operating conditions.
05
05
模型驗證
Model validation
根據(jù)系統(tǒng)故障預(yù)測的仿真,可以驗證維護和維修策略的可行性,并將論證結(jié)果導(dǎo)入策略庫中作為方案。
Based on the simulation of system fault prediction, the feasibility of maintenance and repair strategies can be verified, and the demonstration results can be imported into the expert strategy library as a solution.
06
06
模型部署
Model deployment
部署預(yù)測性維護算法模型,根據(jù)工況識別數(shù)據(jù)的反饋信息進行故障診斷,決定設(shè)備或系統(tǒng)的維修策略;根據(jù)現(xiàn)場工況的數(shù)據(jù)進行多維度分析進行壽命預(yù)測,決定設(shè)備或系統(tǒng)的維護和保養(yǎng)策略。
Deploy predictive maintenance algorithm models, diagnose faults based on feedback information from condition identification data, and determine maintenance strategies for equipment or systems; Perform multi-dimensional analysis based on on-site working conditions data to predict service life and determine maintenance and upkeep strategies for equipment or systems.
為解決焊接機器人規(guī)?;瘧?yīng)用過程中操作與維護規(guī)范化問題,通過分析焊接機器人應(yīng)用現(xiàn)狀,應(yīng)用意義及發(fā)展前景,展現(xiàn)焊接機器人操作與維護規(guī)程必要性,同時分析焊接機器人在日常應(yīng)用中存在的不足及問題,突出焊接機器人操作及維護規(guī)程的重要性。更多相關(guān)事項就來我們網(wǎng)站http://niuniury.com咨詢!
To address the standardization of operation and maintenance in the large-scale application process of welding robots, the necessity of welding robot operation and maintenance regulations is demonstrated by analyzing the current application status, significance, and development prospects of welding robots. At the same time, the shortcomings and problems of welding robots in daily applications are analyzed, highlighting the importance of welding robot operation and maintenance regulations. For more related matters, come to our website http://niuniury.com consulting service